Data Branching for Batch Job Systems
Data is being increasingly treated like code has been treated for decades. For many use-cases it isn't enough to know "What is the current value?" but also "What was the value previously?", "Who last changed the value?", and "Why did they change the value?".
Having a history for data provides data security benefits by always being able to rollback to a previous value (and not just the last value, but any preceeding value). It provides an audit trail that can capture a lot more of the "Why" of data changes than purely "Person/System X changed the value at datetime Y". This can help with debuggability of data processes.
These benefits are behind the invention of tools such as lakeFS (2020) and Oxen.ai (2022). Both build out a Git-for-data system, involving the creation of data repositories, data branches, commits, merge commits, pull requests, etc. Planetscale has even been doing this for SQL databases. But how should these tools be used with a job-based batch data platform?
Branches for Jobs
The "main" branch should be considered the canonical, production version of data. At the start of each job execution we can branch off this main branch and create a branch for our job execution.
This provides a safe place where our job can write its data (potentially in multiple steps), record all the metadata it needs to, and then decide whether to merge back into the main branch (such as if the job succeeded overall).
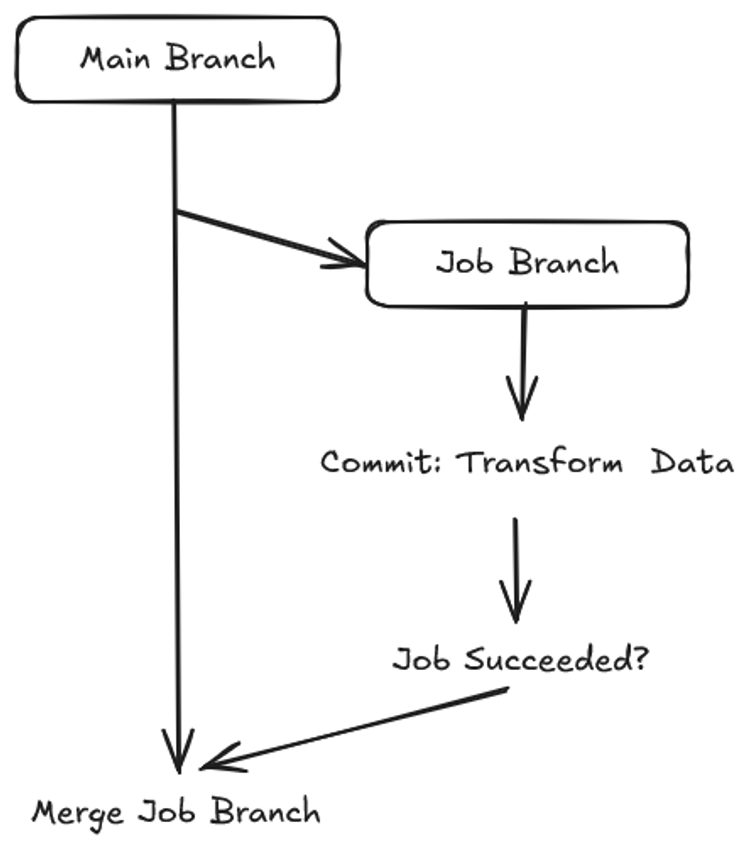
Branches for Test Executions
It can be valuable to run tests (manual or automated) with production data as an input, but without the risk of affecting production data on the output. We can use branches to provide this guarantee if we simply discard our job branch at the end of the execution.
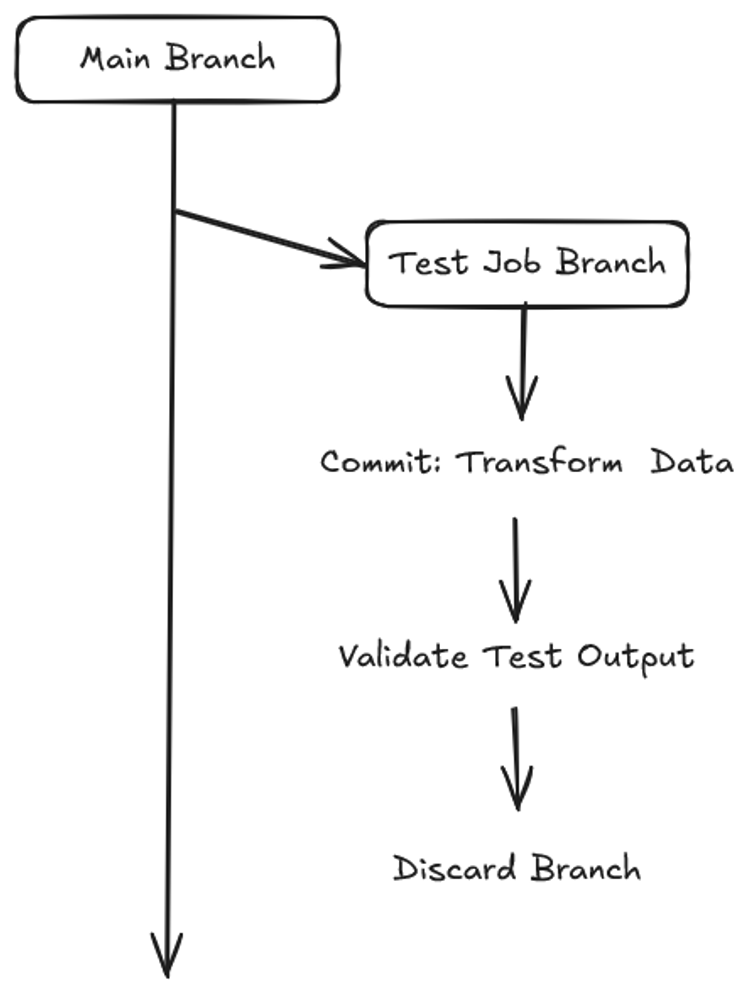
Branches for Experiments
For cases where we want to keep experimental data around for longer (i.e. not discard the output after a single job execution, but also not merge it to main) we can make use of experiment branches. These branches are longer lived than job branches, but are not the canonical version of data in the repository.
These branches can be used to execute multiple jobs back-to-back on experimental data, such as passing the output of one experimental job to another experimental job - allowing for data experiments that cover multiple steps of a data pipeline.
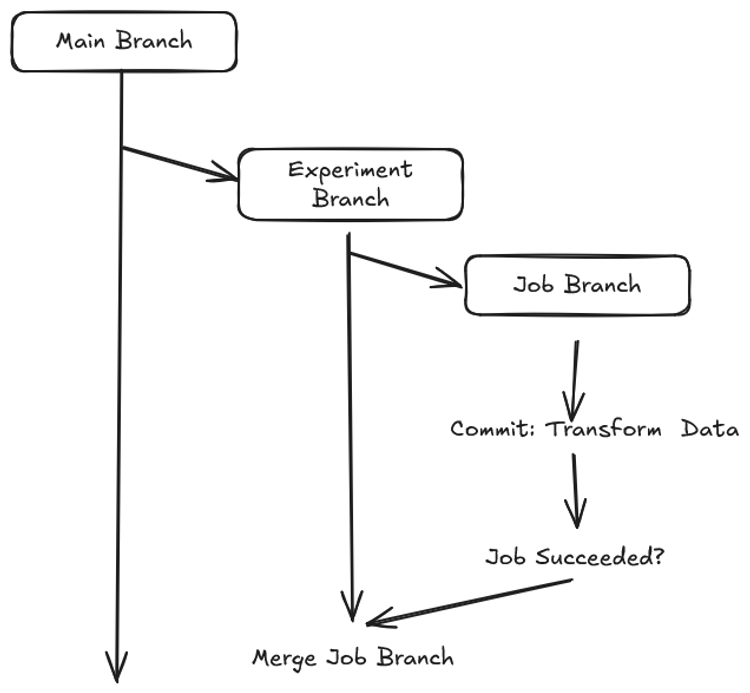
Branches for Multi-Step Jobs
In the case that our job is particularly complex and needs broken down into multiple, potentially parallelisable operations, it can be useful to create branches for each of these operations. In LakeFS and Oxen.ai this provides separate staging areas for each operation allowing each operation to commit its own changes and only its own changes at the end of the operation.
After all operations are complete, the job branch can be tidied up and evaluated as to whether the data should be merged back into the main branch - to become the new canonical version of the repository's data.
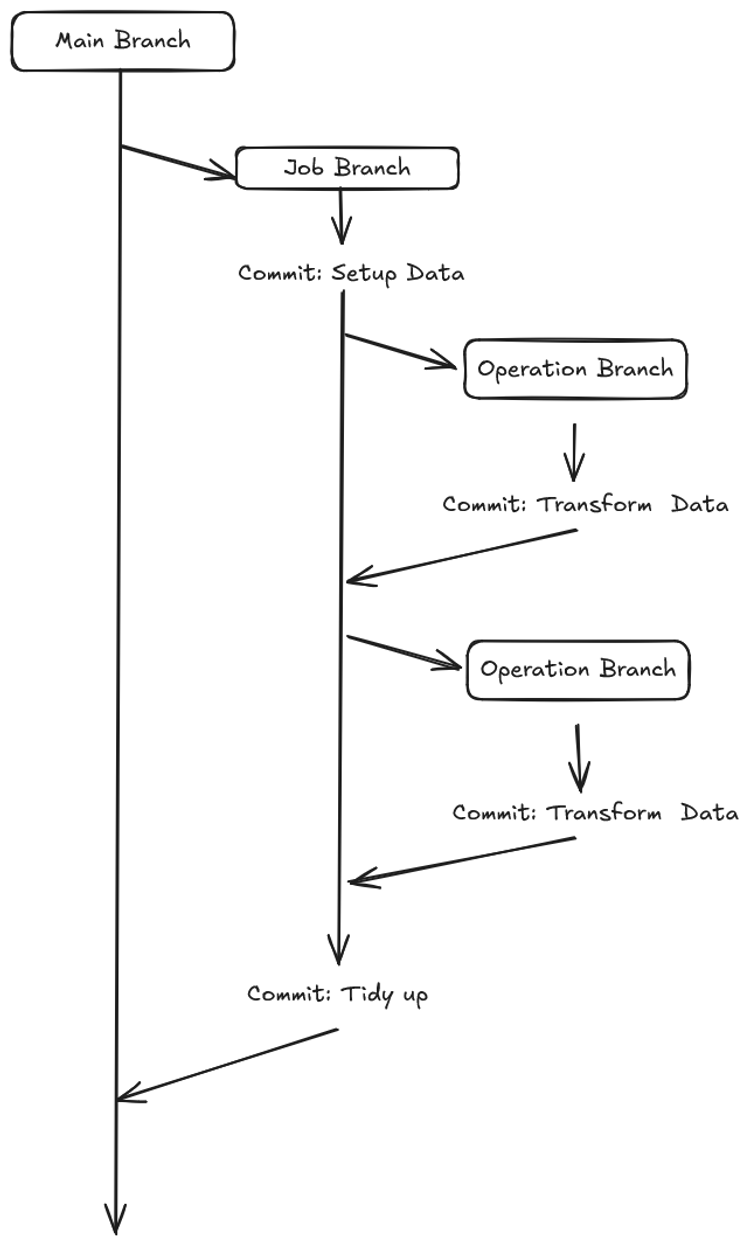
Conclusion
In this blog post I've shown how I've been thinking about data branches for different use-cases in a batch job-based software system. In LakeFS and Oxen.ai, creating new branches is a very cheap operation as it doesn't require creating a copy of the existing data. This allows us to create staging areas for data to be written or modified, while deciding at the end whether to apply these modifications to our canonical production data. In some ways, these branches end up looking a lot like database transactions with certain ACID guarantees.
I'm sure there's more sophisticated patterns out there, so if you've got any feedback or suggestions please reach out!
If you enjoyed this post, please check out my other blog posts.